Zirui's Homepage
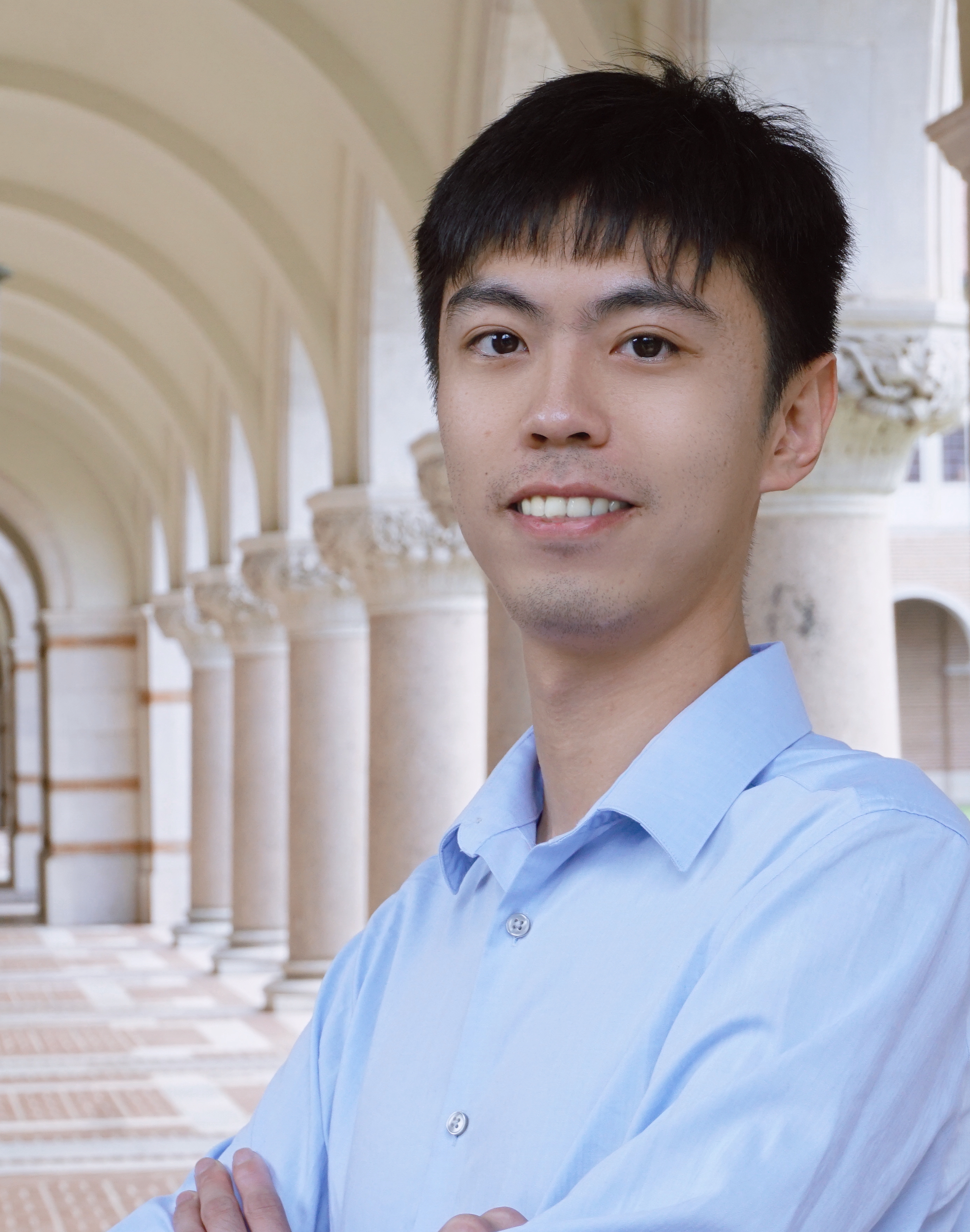
Duncan Hall, 3014
6100 Main St
Houston, TX 77005
About me
I’m Zirui “Ray” Liu, an incoming assistant professor from the Department of Computer Science at UMN. Previously I graduated from Computer Science at Rice University, co-advised by Dr. Xia “Ben” Hu and Prof. Vladimir Braverman.
My core research interests lie in large-scale machine learning and machine learning system. I co-design algorithm and system, aiming to scale-up and/or accerlate machine learning models. Some examples include LLM KV Cache quantization, randomized matrix mulplication, and GNN quantization. Besides MLsys, I am also interested in other core problems/applications in LLMs like RAG, long context ability, and LLM safety.
Feel free to reach out if you would like to collaborate on MLSys or on-device ML research.
📧📧 Recruiting 2025 Spring/Fall Ph.D. students and interns: I am looking for self-motivated students with strong background in HPC, NLP, and ML. Feel free to drop me a line to ziruiliu dot recruit at gmail dot com together with resume and transcripts if you are interested.
News
-
🔥🔥 Our KIVI largely inspires the KV Cache quantization system in Huggingface. Code is available here; And our Self-Extend is highlighted during Google I/O session. Code is available here.
-
Our KIVI, Self-Extend, and Compress-then-prompt are accepted by ICML 2024.
-
Our Memory-Efficient LLM fine-tuning work is covered by Rice CS New.
-
Three paper accepted to Neurips 2023.
-
Two paper accepted to TMLR.
-
Twos papers accepted to ICML 2023.
-
One paper accepted to MLSys 2023.
-
Two papers accepted to Neurips 2022, DreamShard and GNN Benchmark (Benchmark track).
Publications
Please refer to publications or Google Scholar.